Intention-aware Collision Avoidance
Active Learning-augmented Intention-aware Obstacle Avoidance of Autonomous Surface Vehicles in High-traffic Waters
This paper enhances the obstacle avoidance of Autonomous Surface Vehicles (ASVs)
for safe navigation in high-traffic waters with an active state estimation of
obstacle's passing intention and reducing its uncertainty.
Our main contributions are:
(a) topological modeling of passing based on maritime navigation's
inherent conceptual topolog and implementation of LSTM-backbone-based intention classification
(b) a novel multi-objective local planner that includes an active strategy
to increase information gain in uncertain encounters about the passing intention
of obstacles, while ensuring collision avoidance; and
(c) implementation in Robot Operating System (ROS) with comprehensive analysis
through extensive Monte Carlo simulations, experiments in the ocean with a real ASV,
and a real-world accident case study successfully demonstrating safe and real-time collision avoidance.
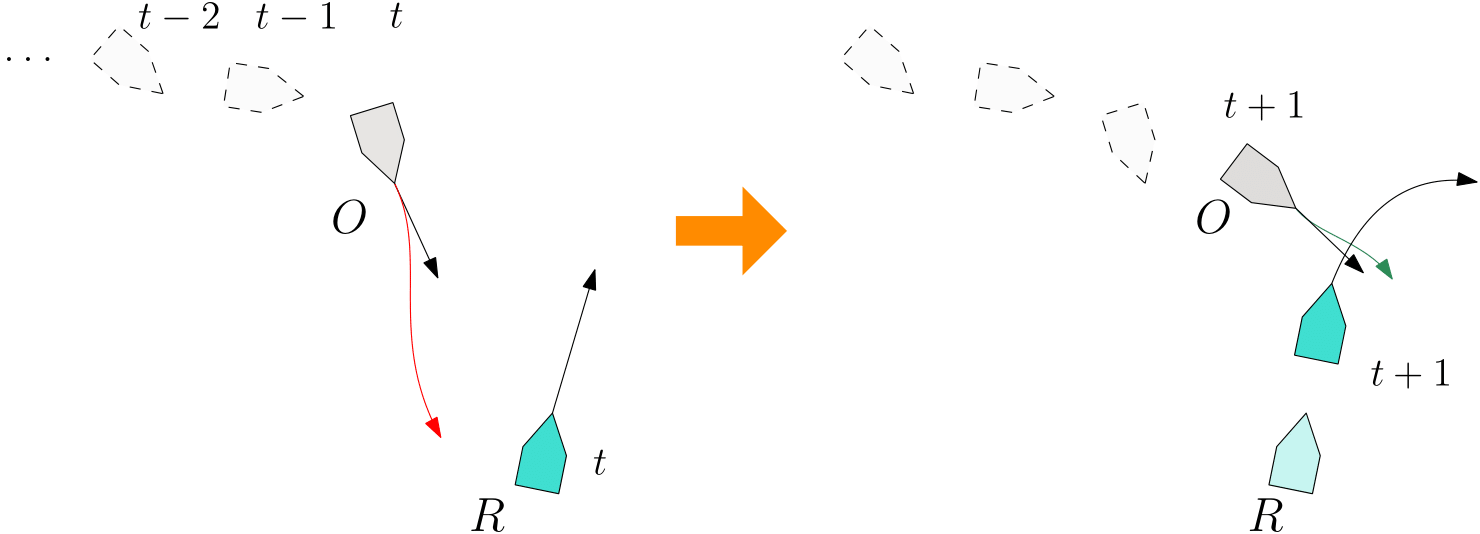
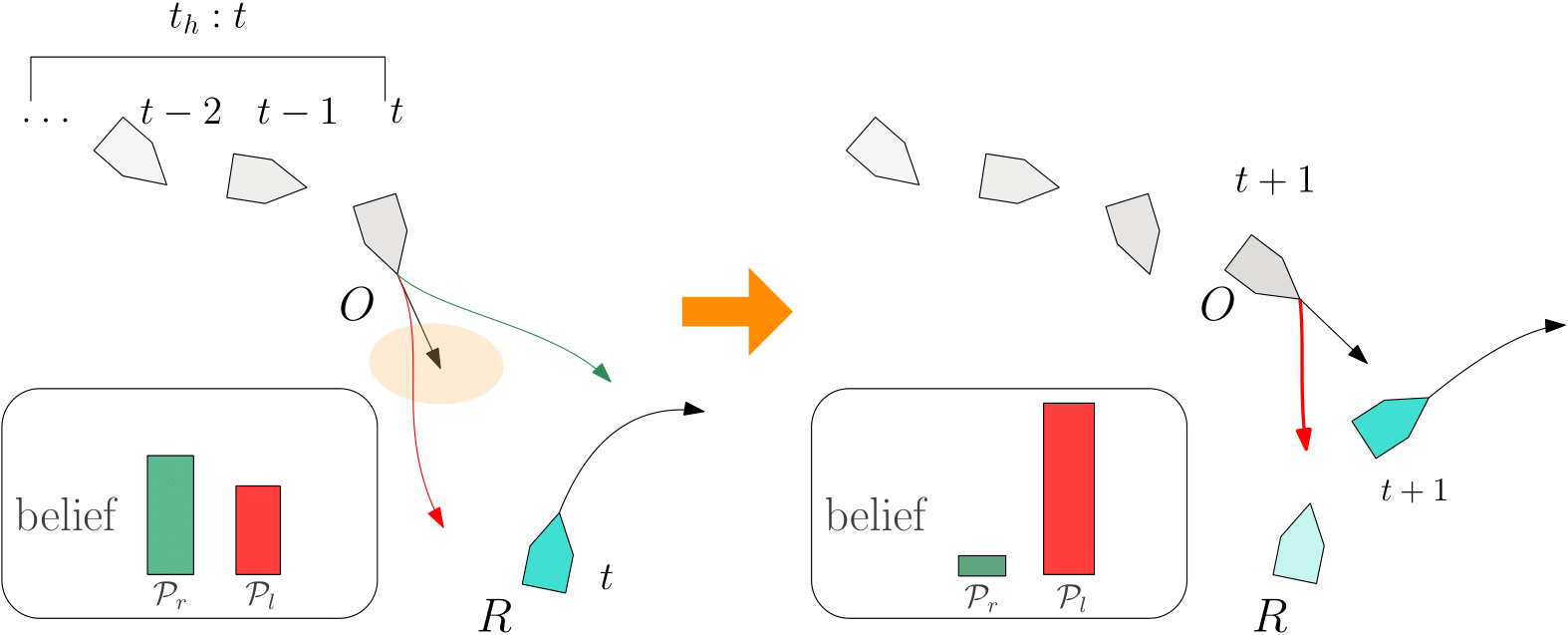
Controlled ASV, R's collision avoidance by state-of-the-art method vs.proposed method using active learning-augmented intention-awareness under an uncertain scenario where an obstacle, O approaches from the left side of R.
Here is a video I have presented at IROS2024.